Resources / The Evolution Blog
Sharing Intelligence Without Compromising Customer Privacy
Rebekah Moody
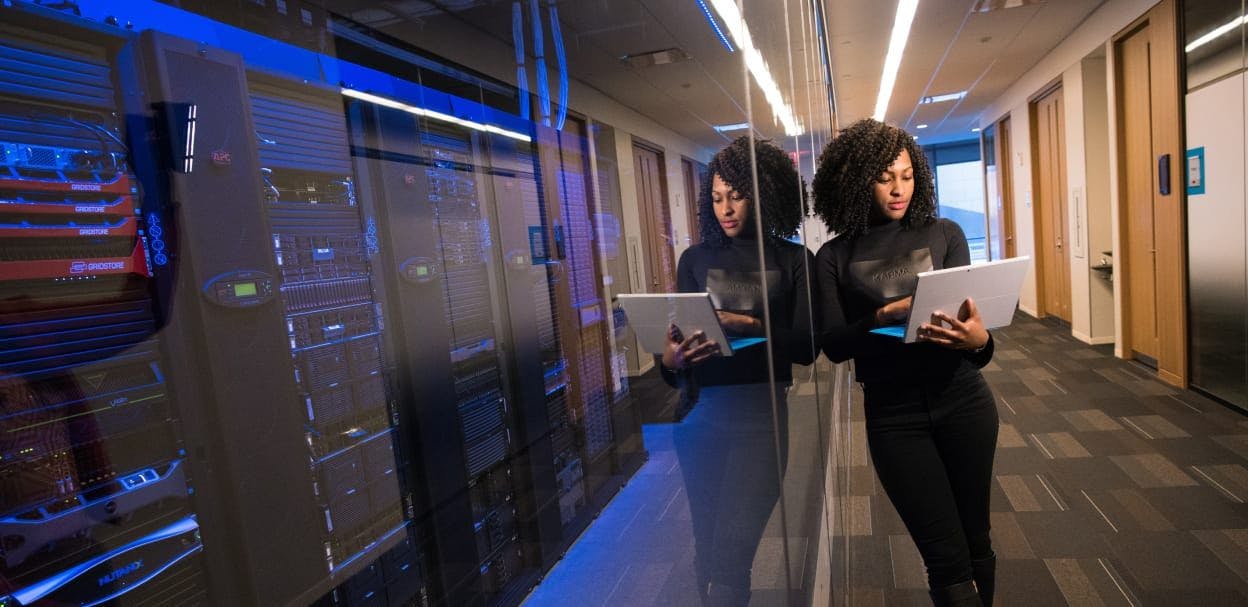
The Evolution Blog Series: Privacy
How to leverage the latest Privacy Enhancing Technologies (PETs) to facilitate intelligence sharing without compromising customer privacy.
Privacy Enhancing Technologies (PETs) encapsulate the next generation of tools, techniques and encryption methods that help digital and security teams better meet the competing demands of digital risk automation, privacy, regulatory compliance and customer experience. They seek to minimize personal data use while maximizing data security, allowing different parties to access data intelligence without relying on mutual trust.
According to Gartner®: “As workloads rapidly shift to the cloud, privacy enhancing computation methods are emerging to enable enhanced confidentiality and privacy in cloud environments, while breach and attack simulation tools facilitate the defense of these complex security stacks with continual security testing”.*
As regulations evolve to prioritize the needs and preferences of end consumers, innovations that lead the privacy race now will no doubt evolve to become industry standards.
Lunar Ventures observes that “for digital ecosystems to reach their full potential, we will need data sharing to be simpler and baked right into the software itself…. PETs enable multiple parties to collaborate on the same data without falling foul of regulators or increasing risk by leaking personal data”.**
Examples of current PETs that are facilitating new data and intelligence sharing use cases include:
- Zero Knowledge Proofs: One party can prove to another party that they know a particular value within a dataset, without revealing what the value is. This allows the data to be audited without revealing any personal / underlying information.
- Homomorphic Encryption: In this scenario, the data controller does not need to trust the party receiving the encrypted data. Data can be processed or analysed without decrypting it, removing the risk associated with sharing data intelligence. Different parties can therefore share and interrogate intelligence relating to the same dataset.
- Polymorphic Encryption: This allows different parts of a dataset to be shared with different parties, i.e. the encryption and decryption pair can change every time they are used. This means that the data controller can choose which parts of a dataset to share with which user, depending on what they need access to.
- Synthetic Data Generation: Harnessing a created set of data that closely mimics or reflects a real dataset can allow various critical business cases to be tested and refined. While the integrity of the real data is maintained, the synthetic dataset can be expanded to allow advance machine learning and artificial intelligence experimentation and shorten timeframes to market. This approach facilitates baselines of user and attacker behavior to be set, as well as attack simulation to test detection systems and responses.
- Federated Machine Learning: Contrary to traditional machine learning techniques that bring datasets together, federated machine learning can train an algorithm across multiple decentralized datasets. Multiple parties can therefore harness the same machine learning model without sharing data.
While protecting customer privacy in cloud environments is paramount, businesses are also increasingly relying on the democratization of data and intelligence sharing both internally and externally for a wide variety of reasons:
Sharing Data Within Organizations
Within disparate business units or companies that have merged through acquisition, the ability to share customer intelligence or elements of customer data in a secure and private way is often critical. This challenge can sometimes be compounded across different regional business nodes when regulatory environments impose different restrictions on the handling of personal data.
Sharing Intelligence Across Networks of Different Businesses
For many fraud, anti-money laundering and risk intelligence use cases, sharing intelligence between organizations is critical to expose the ever-more complex networks of money mules and global fraud rings. This may incorporate sharing intelligence across multiple law enforcement agencies and the global banking ecosystem.
PETs allow the protection and privacy of both data at rest and data in motion to ensure businesses can evolve to meet new and complex business challenges.
Key Privacy Principles
While uncertainty remains around which technologies will lead this arms race to providing the gold standard for customer privacy, several key principles are helpful to consider when evaluating the next generation of security and risk solutions. These can help businesses better evaluation how they can meet complex and evolving privacy considerations both now and in the future:
- Look for solutions that layer multiple PETs within the architecture of a platform to ensure that privacy is built into workflows from profiling, through to testing, deploying, decisioning and offline analytics.
- Allow end users greater control over how their data is used. Consider moving risk decisions closer to the customer’s device to ensure that personal data is only shared when absolutely necessary.
- Ensure that data sharing use cases leverage encryption techniques that preserve the privacy and integrity of the source data so that only intelligence, and not personal information, is shared
- Consider the use of synthetic datasets to simulate user behavior and attack profiles, and to perform complex data analysis on created data that is statistically representative of your raw dataset.
- Consider alternatives to on premise solutions that facilitate private cloud implementations. This approach means that you can maintain complete control of customer data and data residency while enjoying the benefits of cloud-native solutions.
Privacy Becomes Standard
As companies upgrade their fraud, risk and security analytics stack to take advantage of new technologies, privacy will be a key differentiator. Solutions that are privacy-enhanced will enable new and innovative use cases that expand and democratize the way that data can be used without compromising customer privacy. Over time this will push privacy enhancing principles to be incorporated as standard, driving significant incremental value above legacy solutions.
About Darwinium
Darwinium is a Digital Risk platform that harmonizes fraud and risk with customer experience and digital security. Darwinium can be deployed as an Edge worker via your CDN, or as an NGINX plug in.
Darwinium manages the complexity of making real-time decisions on customer data, and provides encryption and masking controls over any data extracted and shared with downstream services. Darwinium is built with PETs as standard, removing the need to send data outside of private boundaries.
Sources
* Gartner, “Top Security and Risk Management Trends 2021”, Peter Firstbrook, Zaira Pirzada, 30 March 2021. GARTNER is a registered trademark and service mark of Gartner, Inc. and/or its affiliates in the U.S. and internationally and is used herein with permission. All rights reserved.
** Privacy Enhancing Technologies: Lunar Ventures: Insight Series. Lawrence Lundy-Bryan